25 Data Science Interview Questions & Answers
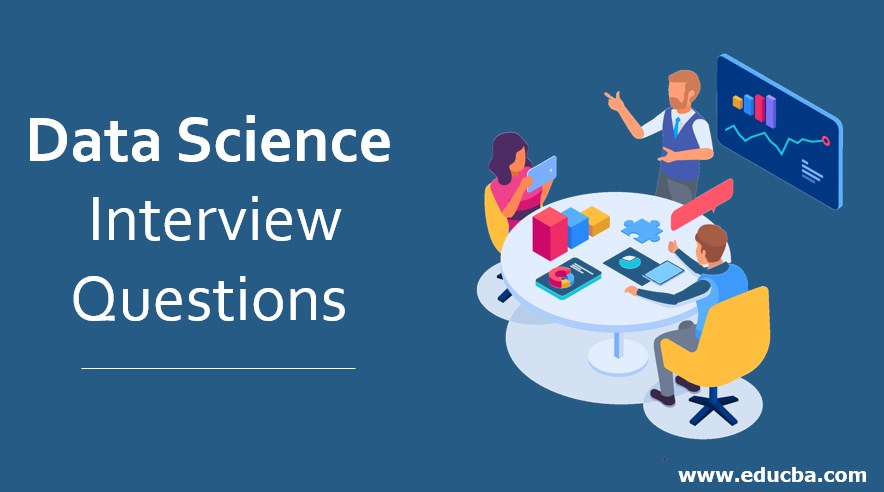
In this article Data Science Interview Questions and Answers, we are adding some specific questions for your interview, we hope you like our article, if you are searching for some interesting off-topic to read then we hope you like this article’s double meaning question. As the world keeps on developing into a time of huge data, there is a fast expansion in the necessity for its capacity. Till 2010, it stayed the essential obstruction and wellspring of vulnerability for the business. The essential areas of the center were the foundation of a structure and the improvement of techniques to store data. Since Hadoop and different structures have effectively handled the test of capacity, the center has changed to the investigation of this data. Data science is the solution that opens these secrets.
With data science, the ideas that are all displayed in Hollywood science fiction films are genuinely fit for being changed into a reality. The field of data science is where computerized reasoning is going soon. Thus, it is of utmost significance to understand what data science is and the way that it might offer worth to an association. To assist you with looking for a way to improve on significant subjects, here is a thorough rundown of data science interview questions.
data science interview questions
If you aspire to become a Data Scientist then you must read these Data Scientist Interview Questions. Let us start with the basic Data Science Interview Questions and then move to the advanced level.
1. Data Science Interview Question – What can you tell us about “Deep Learning”?
Profound learning is a subset of man-made consciousness and AI which utilizes profound brain organizations. These are calculations, almost displayed after the human minds, to educate PC frameworks to duplicate human cerebrums regarding thinking and learning. This is achieved by training PCs to learn in a way like how human minds learn.
Rather than additional traditional ways to deal with AI, profound learning models can hold millions or even billions of boundaries. This makes them more challenging to break down however gives a critical benefit in fathoming data. A profound learning model can be viewed as a “multi-dimensional image” of data since it stores loads. So they are interrelated and reflect bits of what the model has realized during the time spent profound learning.
2. Data Science Interview Question – Explain the distinctions between large data and data science.
Data science is a multidisciplinary discipline that spotlights the insightful components of data and consolidates standards from measurements, data mining, and AI model. These basics are the structure impedes that data researchers use to develop dependable speculations in view of experimental proof.
Huge data works with an immense assortment of data sets and attempts to settle difficulties associated with the administration and handling of data for more educated navigation.
Learn more:- How does imagination play a role in creativity
3. Data Science Interview Question – Explain the regression data set.
The relapse data set is a reference to the registry containing the test data for the straight relapse model, known as the data set catalog. The most essential sort of relapse is deciding the best straight association between a given arrangement of data (xi, yi) that has been gathered.
4. Data Science Interview Question – Why data cleansing is necessary?
The course of “data purging” includes figuring out all of the data contained in a database and eliminating or refreshing any data that is viewed as mistaken, fragmented, lacking, or over the top. Doing so upgrades the dependability of the data, which is of basic significance.
5. Data Science Interview Question – What is meant by the term “machine learning”?
AI is a subfield of software engineering that utilizes numerical calculations to find the pattern or example in a whole dataset. The expression “AI” comprises two words: machine and realizing, which indicate what it is.
The most rudimentary delineation of this idea is straight relapse, which is addressed by the situation “y = mt + c” and is utilized to gauge the worth of a solitary random variable y as an enactment capability of time. By applying the condition to the data in the set and figuring out which values for m and c produce the best fit. The AI model can find out about the examples in the data. From that point forward, one can use these conditions and perplexing factors to assess what’s in store values will be.
6. Data Science Interview Question – What do you know about recommendation systems?
Acquiring an understanding of the way of behaving of clients and potential clients is a significant focal point for many firms. Ponder what is happening with Amazon, for instance. Amazon’s backend grouping calculations have a huge obstacle at whatever point a client looks for an item class on the organization’s site: the objective is to create ideas for such clients that truly are probably going to urge them to make a buy.
Furthermore, these characterization calculations give the fundamental part of suggestion frameworks, frequently known as recommender frameworks. These frameworks are intended to dissect clients’ way of behaving and decide the degree of adoration clients have for different items. Recommender frameworks are used by different web-based retailers, including but not restricted to Netflix, YouTube, Flipkart, and others, notwithstanding Amazon.
7. Data Science Interview Question – What is the difference between an “Eigenvalue” and an” Eigenvector”?
While endeavoring to understand straight changes, eigenvectors are an important device. They are likewise the bearings where a particular direct change acts by either flipping, packing or extending the data. The speed of the shift in the eigenvector’s course is known as the eigenvalue, which can likewise be considered the component by which the pressure happens. In data examination, registering the eigenvectors of a relationship or covariance network is a common practice.
8. Data Science Interview Question – Could you please explain feature vectors?
The assortment of ward factors inside a whole dataset that contain values portraying the characteristics of every perception is alluded to as a component vector. These vectors are utilized as info vectors for a factual model that uses AI.
9. Data Science Interview Question – What is meant by the term “Regularization”?
Regularization is a technique used to push or invigorate the coefficients of the logit model of AI or profound learning toward zero to dispense with the issue of over-fitting. This is achieved using a procedure known as “push-learning.” As an expansive idea, regularization plans to work on complex models by making them harder to tackle by remembering an extra punishment for the misfortune capability that makes it produce a more prominent misfortune. Along these lines, we can keep the model from obtaining exorbitant detail, which brings about the factual model having a fundamentally more broad understanding.
10. Data Science Interview Question – What does “P-value” signify?
In measurements, the p-esteem is a strategy for deciding if the invalid speculation has any huge bearing on the data. A p-esteem that is under 0.05 demonstrates that there is under a 5% likelihood that the consequences of a randomized trial are random, showing that the invalid speculation ought to be dismissed. Then again, if the p-esteem is more noteworthy, say 0.8, it shows that the invalid speculation can’t be dismissed in light of the fact that 80% of the example comprises people who experienced random results.
11. Data Science Interview Question – Could you explain the concept of the normal distribution in its standard form?
In the field of measurements, a remarkable kind of ordinary circulation known as the standard typical conveyance is described by a mean worth of nothing and a standard deviation that rises to one. As per the customary definition, the diagram of ordinary dissemination seems to be the notable ringer bend with no in the center of it. As should be visible, the likelihood of appropriation is entirely even around the starting place, and it shows no side effects.
12. Data Science Interview Question – What is “the curse of dimensionality”?
The expression “high layered data” alludes to data with a critical number of principal traits. How many unmistakable elements or qualities are contained inside the data is alluded to as the component of the data. The expression “revile of dimensionality” alludes to the hardships that manifest themselves while working with data that has countless aspects. More or less, it basically implies that the extent of the slip-up will increment according to the number of highlights present in the data. High-layered data can, in principle, hold more data than lower-layered data.
In any case, by and by, this isn’t useful on the grounds that high-layered data could have more significant levels of clamor and overt repetitiveness. While working with high-layered data, making characterization algorithms may challenge. Furthermore, how much time is expected to finish the job increments dramatically with the quantity of data aspects?
13. Data Science Interview Question – Why do we do “A/B” tests, and what do we want to accomplish with them?
The A/B test is a sort of factual speculation testing intended for use in a randomized trial including two different jumbling factors, An and B. The reason for A/B testing is to decide if a page has been changed in any capacity, with the end objective of expanding the chance of a result that is of significance to the member.
A/B testing is an entirely reliable methodology that can be utilized to decide the most compelling internet-promoting and publicizing strategies for simply an organization. This strategy can be utilized to test anything, from deal messages to web ads and site duplicates, and to apply to these areas.
14. Data Science Interview Question – Can you explain the difference between linear regression and logistic regression?
A kind of measurable technique known as the direct relapse model is one in which the worth of a specific random variable, signified by the letter Y, is anticipated in view of the worth of a subsequent variable, signified by the letter X, and alluded to as the indicator variable. The letter Y means the measures random variable, which is available in the situation. The straight relapse model aids in deciding the upsides of specific data and spotting the example.
Calculated relapse is a measurable methodology for anticipating the double outcome utilizing just a straight blend of indicator subordinate factors.
15. Data Science Interview Question – What is the meaning of the term “dropout”?
In the field of data science, the expression “dropout” alludes to a procedure utilized to randomly eliminate units from an organization, both covered up and obvious. By eliminating upwards of a fifth of the hubs from the organization, they stay away from overfitting the data and make it conceivable to orchestrate the essential space for the emphases expected to combine the organization.
16. Data Science Interview Question – What is meant by the term “cost function”?
A model’s exhibition can be assessed utilizing cost capabilities to decide how well the logit model is doing. It considers any deficiencies or misfortunes that happened in the result layer while the back-engendering is done. In a situation like this one, the remaining mistakes are moved in the brain network the other way, and different other preparation piece capabilities are carried out.
17. Data Science Interview Question – Could you please explain the meaning of hyperparameters?
Hyperparameter is a kind of boundary, and its worth is resolved even preceding the growing experience begins. This is to ensure that the organization preparing necessities not entirely set in stone and the design of the organization might be gotten to the next level. This additionally incorporates distinguishing things like secret units, learning rates, and ages, in addition to other things.
18. Data Science Interview Question – Could you please explain the concept of batch normalization?
Group standardization is a method that empowers us to upgrade the strength and productivity of a brain organization. Such enhancements could be utilized in this method. This should be possible by standardizing the contributions for each resulting layer. In this way, the resulting enactment keeps on being zero while the standard deviation is kept at one.
20. Data Science Interview Question – What are tensors, and how do they work?
Tensors are numerical substances that mirror the collection of additional dimensionality of data inputs in letters in order, numerals, and a position that are given as contributions to a brain organization. All in all, tensors are the assortment of higher layered data inputs.
21. Data Science Interview Question – What is the activation function?
The actuation capability works with the presentation of non-linearity into the brain organization. With regards to learning convoluted portion works, this is finished to assist with making the educational experience simpler. Without the actuation capability, the brain organization will not be able to work the straight initiation capability exclusively or execute direct blends. The actuation capability thusly offers confounded portion capabilities and blends by utilizing fake neurons, that help with offering a yield in view of the data sources. This is made conceivable by the utilization of counterfeit brain organizations.
22. Data Science Interview Question – Could you please explain RNN to me?
“Intermittent Brain Organizations” are a sort of counterfeit brain network comprised of a succession of data. This data grouping could incorporate things like time series, securities exchanges, and different things. The primary point of the RNN application is to analyze the essentials behind feedforward nets.
23. Data Science Interview Question – What is meant by the phrase “reinforcement learning”?
In the AI model, the solo learning method referred to as support learning is known as support learning. This unaided learning is likewise called a state-based learning approach. During the preparation stage, the framework will actually want to progress starting with one state and then onto the next since the models incorporate standards for changing states that have previously been set in unaided learning.
24. Data Science Interview Question – Explain selection bias.
Determination predisposition is the cycle when there is no random assortment of examination members. How the random example is gathered can bring an inclination into measurable examination, which can then be viewed as having been misshaped subsequently. The expression “determination impact” can at times be utilized reciprocally with “choice predisposition.” Assuming specialists neglect to represent potential inclinations in the determination of study subjects, the consequences of their examination may not be dependable.
25. Data Science Interview Question – What are the support vectors in SVM?
Support vectors are data guides that are arranged in nearer vicinity toward the hyperplane and meaningfully affect the position and direction of the hyperplane. We can build the edge of such a classifier by utilizing these help vectors. The area of the hyperplane shifts assuming the help vectors that are currently there are eliminated. These are the contemplations that help us in the development of our SVM.
26. Data Science Interview Question – What is meant by the phrase “root cause analysis”?
The method involved with following back an occasion’s event and the conditions that lead to that belief is known as underlying driver examination. It is ordinarily completed if a piece of programming encounters an issue. Main driver examination is a method utilized in data science that empowers organizations to more readily fathom the implications related to specific outcomes.