Best GPU for Deep Learning: Considerations for Large-Scale AI
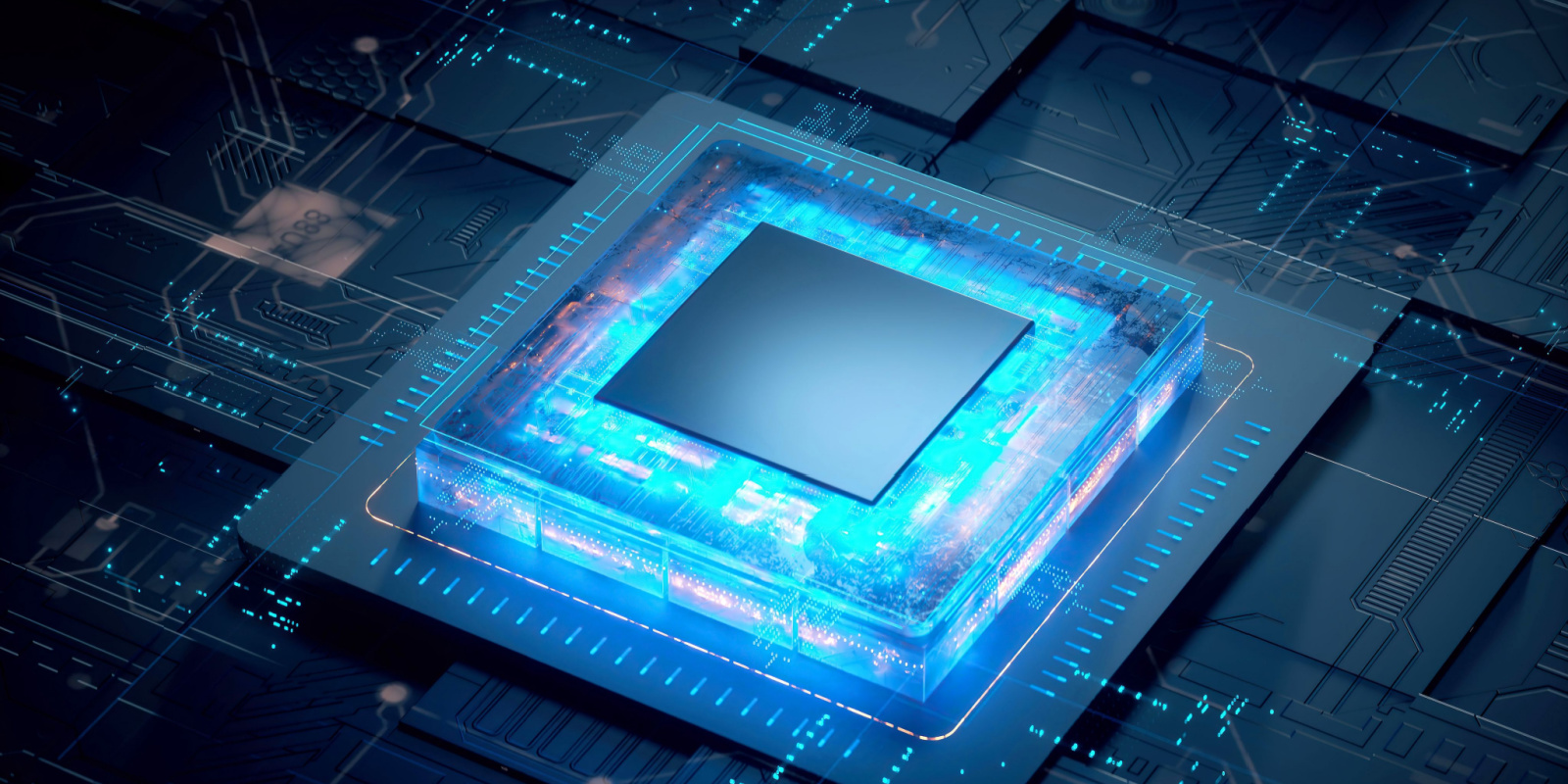
Are you looking to build your own deep-learning system? With the increased demand for Artificial Intelligence (AI) applications, the right GPU cloud is essential for leveraging high-performance computing.
But with so many different GPUs on the market, how can you choose one that best suits your project’s requirements? In this blog post, we’ll dive into the factors such as cost and performance that should be taken into consideration when selecting a Graphics Processing Unit for large-scale AI applications. It will help you decide the best-suited GPU for deep learning.
Read on to discover all you need to know about getting started with cutting-edge machine-learning technology!
Why is GPUs Well-Suited for Large-scale AI?
GPUs are graphics processing units traditionally used for displaying images on a computer screen. However, in recent years, GPUs have been used to accelerate deep learning algorithms and have proven to be very effective in training deep neural networks.
GPUs are very efficient at processing data in parallel, so they are well-suited for AI applications requiring massive data processing. The more data to be processed, the more effective a GPU will be. Along with parallel processing, GPU clouds can provide high throughput.
Now that we know that a GPU cloud is critical to carry out a large-scale AI application and complex computations, it’s essential to know the factors on which you should select the right GPU.
Five Factors to Consider When Selecting a GPU for Large-Scale AI Applications:
When selecting a GPU for large-scale AI applications, it’s critical to focus on a few aspects to get the best solution at a competitive price. The following factors are crucial and should be considered when selecting a GPU cloud for AI apps:
1: The Size and Complexity of the Neural Network Model
The size and complexity of the neural network model matter because they affect the number of degrees of freedom (parameters) in the model. It, in turn, affects the ability of the model to learn and generalize from data. For instance, a complex neural network has many interconnections between neurons and can better know intricate patterns in the data. A simple neural network has fewer interconnections and is not as good at learning complex patterns, but it is easier to understand.
Similarly, a more extensive neural network has more parameters and is better able to learn specific details about the training data. A smaller neural network has fewer parameters and can better generalize from data but may need more accurate predictions for large-scale AI applications.
2: The No. of Neurons in the Network
Since there are many different types of AI applications (each with its own set of requirements), knowing the correct number of neurons can be a tough nut to crack. However, a recent study by Google found that a network of approximately 16,000 neurons can be used to achieve near-human accuracy. It’s important to note that this number may vary depending on the specific application and the accuracy required.
For example, networks with more neurons may be needed for tasks requiring greater precision or large-scale AI applications that need to operate in real-time. Conversely, smaller networks may be sufficient for more straightforward tasks or require less precision.
3: The Amount of Data to be Processed
GPUs can handle large datasets much faster than CPUs because thousands of cores operate in parallel. This parallelism allows them to divide the workload and process it faster than a CPU. In addition, GPUs include dedicated hardware called “stream processors” designed explicitly for matrix operations, which are essential for deep learning and other AI applications.
Thus, an advanced GPU cloud such as NVIDIA A100 GPU is the right choice as it can handle large amounts of data and process them parallelly like a breeze.
4: The Type of Training Algorithm
What the right kind of training algorithm is – It heavily depends on the specific application at hand. Several GPU training algorithms are particularly well-suited for large-scale AI applications. One such algorithm is the deep learning algorithm, which has demonstrated impressive performance gains in recent years across various domains, including image recognition, natural language processing, and machine translation.
Another popular GPU cloud training algorithm is the Recurrent Neural Network (RNN), which has been shown to be effective in handling long data sequences, such as speech recognition and handwritten character recognition.
5: The Type of Hardware Platform
While there is no one-size-fits-all regarding the best hardware platform, a distributed hardware platform like Spark or Hadoop is often well-suited for large-scale AI applications. It is because these platforms enable data parallelism, allowing individual nodes to work on different parts of the data simultaneously. It can be especially beneficial for AI applications, which often require a lot of processing power.
Additionally, hardware platforms by Ace Cloud Hosting are usually very scalable, meaning they can handle large amounts of data without becoming bogged down. It can be crucial for AI applications, which often require a lot of processing power and storage capacity.
Conclusion
Artificial intelligence is becoming increasingly important as we move towards a digital future. As such, it’s crucial to choose the right GPU cloud for deep learning in order to create large-scale AI models efficiently and effectively. And since GPUs can process many calculations in parallel, it makes them ideal for deep learning applications that require much computational power.
Consider factors such as cost, computational power, energy efficiency, memory capacity, and bandwidth when deciding. Ace Cloud provides high-quality GPU clouds that offer excellent computational power and energy efficiency at an affordable price point. Visit Ace Cloud’s website today to learn more about their products, pricing, and their benefits for your business.